Facial Visual Infared Stereo Vision Fusion Measurement for Internal State Estimation
Keywords:
Mental, physiological, stress, SVM, visionSynopsis
Our main aim is to propose a vision-based measurement as an alternative to physiological measurement for recognizing mental stress. The development of this emotion recognition system involved three stages; experimental setup for vision and physiological sensing, facial feature extraction in visual-thermal domain, mental stress stimulus experiment and data analysis based on Support Vector Machine (SVM). In this thesis, 3 vision based measurement and 2 physiological measurement was implemented in the system. Vision based measurement in facial vision domain consists of 3 ROI’s temperature value and blood vessel volume at supraorbital area. Two physiological measurement were done to measure the ground value which is heart rate
and salivary amylase level. We also propose a new calibration chessboard attach with fever plaster to locate calibration point in stereo view. A new method of integration of two different sensors for detecting facial feature in both thermal and visual is also presented by applying nostril mask, which allows one to find facial feature namely nose area in thermal and visual domain. Extraction of thermal-visual feature images was done by using SIFT feature detector and extractor to verify the method of using nostril mask. Based on the experiment, almost 98% match was detected successfully for without glasses and 89% with glasses. Graph cut algorithm was applied to remove unwanted ROI. The recognition rate of 3 ROI’s was about 90-96%. We also presented new method of automatic detection of blood vessel volume at Supraorbital monitored by LWIR camera. The recognition rate of correctly detected pixel was about 93%. An experiment to measure mental stress by using the proposed system based on SVM classification had been proposed and conducted nad showed promising results.
Downloads
References
A. Rosenfeld. (1999). “Image Analysis and Computer Vision: 1998,” Comput. Vis. Image Underst., vol. 74, no. 1, pp. 36–95, Apr.
D. G. Lowe. (2004) “Distinctive Image Features from Scale-Invariant Keypoints,” Int. J. Comput. Vis., vol. 60, no. 2, pp. 91–110, Nov.
M. M. Bundele and R. Banerjee. (2010). “ROC analysis of a fatigue classifier for vehicular drivers,” in 2010 IEEE International Conference on Intelligent Systems, IS 2010 - Proceedings, pp. 296–301.
M. P. Tarvainen, J.-P. Niskanen, J. a Lipponen, P. O. Ranta-Aho, and P. a Karjalainen,. (2014). “Kubios HRV--heart rate variability analysis software.,” Comput. Methods Programs Biomed., vol. 113, pp. 210–20.
R. Fernandez and R. W. Picard. (2003). “Modeling drivers’ speech under stress,” Speech Commun., vol. 40, pp. 145–159.
R. Kumar, D. M. Tullsen, P. Ranganathan, N. P. Jouppi, and K. I. Farkas. (2004) “Single-ISA heterogeneous multi-core architectures for multithreaded workload performance,” Proceedings. 31st Annu. Int. Symp. Comput. Archit.
Y. Fukuoka and A. Ishida. (2000) “Chronic stress evaluation using neural networks,” IEEE Eng Med Biol Mag, vol. 19, pp. 34–38.
Z. Zhu, P. Tsiamyrtzis, and I. Pavlidis. (2008). “The Segmentation of the Supraorbital Vessels in Thermal Imagery,” 2008 IEEE Fifth Int. Conf. Adv. Video Signal Based Surveill., pp. 237–244, Sep.
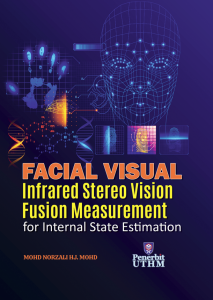